Machine learning (ML) is the tool used to achieve some type of goal in your business. The goal can be increased efficiency with a purpose to cut costs. It can also be the sales growth through a more accurate understanding of your customers’ needs, offering better customer experiences, improving the decision making or leveraging ML so it can help your business to scale. All those goals are very different by nature and require a separate approach – so if your company doesn’t exactly have a huge amount of money to burn, then most probably you don’t want to solve all those problems at once. Hence, you have to decide to achieve which goal helps to create the most value for your business and then proceed with finding ways how to do that.
Saving costs and increasing revenues are the most common, but yet quite general goals everyone’s talking about. In this post, I will focus mainly on how ML helps in scaling your business operations. Scaling is almost like a combination of saving costs and increasing revenues, more precisely it’s handling the significantly increased demand (e.g. from reaching to new markets) through the cost-effective way. Scaling is performed mainly by the startups, which want to “disrupt the world as fast as possible”, but actually it’s more than relevant also to mature businesses. In the end, companies have quite equal opportunities in choosing how to perform their operations – it all comes down to a decision, which doesn’t depend either if it’s a startup or an entity established long time ago. (However, it can be tougher for a mature company to actually pursue that path due to the corporate business structure – check my last post “The Ultimate Battle of AI between corporates and startups”).
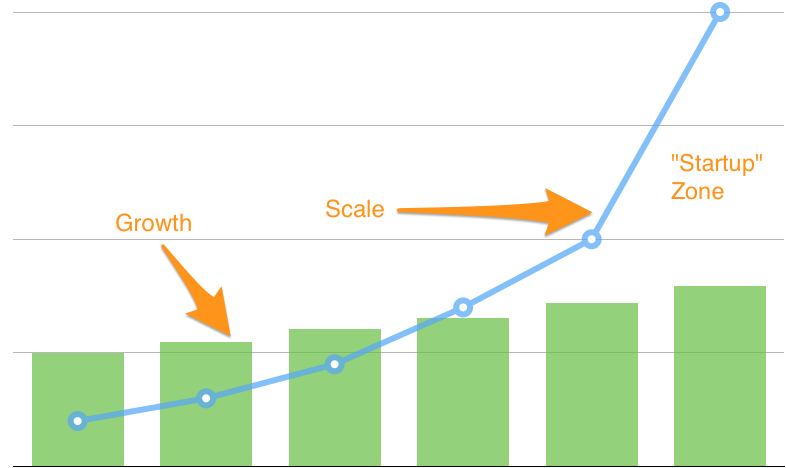
The success wouldn’t have been possible for many very well known companies without the ability to scale and it’s partly thanks to ML. Uber applies ML in predicting the demand and supply for the rides, as well as fixing the price range for customers among many other things. Credit institutions wouldn’t be able to offer reliable and instant credit decisions without machine’s specialties in document processing, fraud detection, and anti-money laundering. International companies with large volumes of customer contacts can automate less value-adding (both to the customer and the company) and easier communications through the usage of chatbots. All those areas are examples in which ML has helped companies to expand without having the need to employ a large number of people. Just imagine how many people would those companies need if they would like to get those things done with the same scale? A data scientist from one credit provider told me once that “We technically could hire tens of thousands people who would review the customers’ loan applications, perform a background check and different fraud procedures… but should we?”
I was recently participating in TransferWise Hackathon 2019, where our team developed an idea of a virtual fitting room to support the growth of e-commerce and decrease the high return rates. The underlying idea was to create a personalized 3D model of the customer’s body based on the body measurements, and then use 3D matching at e-shop’s website to fit the clothes to the model and see how would they show off on the person. We brainstormed quite many ideas on how to solve the process of getting reliable body measurements with user-friendly way and came up with for example such:
- Use a professional tailor for maximum accuracy
- Customer measures the body on her/his own for cost efficiency
- Place measurement machines at shopping malls and physical stores for maximum accuracy and convenience (very expensive!)
- Use image detection for automatic body measurements for maximum user experience
In a traditional business environment, the first two options might seem the most logical ones. However, using professional tailor sets us limits in scaling and is not exactly the most convenient solution for the customers. Them measuring own body is another solution and at the start also quite a good one from a product perspective, but it makes the customer onboarding bit clumsy and might result in very low accuracy due to different measuring techniques. Placing high-tech measurement machines to physical locations might be rise customer awareness, be quite comfortable and very accurate, but requires a crazy large investment and doesn’t allow to scale.
So our fourth option leveraged image detection, which is one of the methods for ML – and it was the one we chose. Our solution required a customer to do only two pictures – one from her/his front and one from the side. It required the customer to insert only their gender and height. Based on that information, the solution covered the picture with data points around the body and calculated all the body measurements, which you could later also modify. There is a company called 3DLOOK, who develops the exact same solution, but they currently only provide you with the matching clothing size (S/M/L, etc.) for that specific shop instead of doing the 3D matching in the virtual dressing room. However, we tested their solution out and it worked really well!
The process was convenient, interesting to use and very fast. The measurements were accurate with +/-1 cm difference maximum if the pictures were done straight from the height of the middle of the body. However, if you do the pictures from too high or low, the measurements can even me +/-10 cm incorrect. Nevertheless, we proved that with nice onboarding the solution provides good user experience with sufficient accuracy, and it allowed us to scale very easily.
Using ML suits very well in situations where the requirements for accuracy aren’t too strict and you can “sacrifice” a bit of accuracy in return to gaining the ability to process large volumes. Of course, it’s important to have high accuracy anyway, but for example, in our situation, we were able to allow some differences as our target mission of enabling the growth of e-commerce and decreasing the return rates was probably achieved with our solution nevertheless.
Machine learning is disrupting how the business is done worldwide. The goal of applying ML doesn’t have to be scaling – it’s only one option. Actually, as you probably understood, there are many different applications of ML (check some of the options at the beginning of the post), but if it comes to scaling your business operations, it definitely helps. My goal with writing this post was to fuel your mind a bit to think about all the possibilities what you could do within your business, independently of what you have done so far. When it comes to ML, there are definitely several options within your company how to apply it and gain benefit. If you are interested in how to benefit from ML but don’t know how to start – I know several of companies who offer consultations in that field (located in Estonia, but offer services globally) and in many cases the first consultation is free. Therefore, don’t hesitate to contact me and ask for recommendations of such companies.